| |
Advanced Fire Detection System Using Infrared
Diagnostics
INTRODUCTION
Future fire detection systems should have the ability of discriminating
signatures between fire and non-fire sources, because nuisance alarm problems
have plagued existing smoke detectors. In high value installations such as
semiconductor clean rooms and telephone central offices, it is obvious that
reliable fire detection systems are needed, since usually these detection
systems are used to activate fixed fire suppression systems, and false
discharges are certainly undesirable. False alarms can cause unnecessary down
time and undermine the operator’s confidence in the monitoring systems.In
light of these, a new fire detection system using infrared diagnostics (FT-IR
spectroscopy) together with advanced signal processing technique (artificial
neural networks) has been developed at Advanced Fuel Research, Inc. This new
fire detection system promises to provide an early warning of hazardous
conditions and has the ability to determine whether the hazardous conditions are
from fire or nuisance/environmental sources.
APPROACH
It has been shown that multi-parameter fire detection systems are inherently
more reliable than any single parameter measurement and can be made robust by
the use of artificial intelligence methods. The objective of our
research efforts is to use an advanced Fourier Transform Infrared gas analyzer
to develop an intelligent fire detection system that can be used in high value
facilities. We have made extensive FT-IR gas measurements of flaming and smoldering fires
as well as environmental/nuisance sources. The FT-IR measurements were made
in open-path, cross duct, and extractive modes for flaming fires, while
measurements of smoldering fires and environmental/nuisance sources were
performed in extractive mode, since most of the current fire detection
technologies (e.g. VESDA and AnaLaser) for cleanrooms and telephone central
offices are based on air sampling techniques in which the air samples from
multiple locations of the rooms are drawn and delivered through an extensive
piping network to a particle analyzer. The FT-IR system can be easily
incorporated in this type of fire detection system, and comparison can be made
with existing technologies.
Numerous materials were tested, including Polyurethane (PU),
Polyvinylchloride (PVC), Polymethylmethacrylate (PMMA),
Polypropylene (PP), Polystyrene (PS), Douglas Fir wood (DF), low
density Polyethylene (LDPE), aqueous Ammonia (NH3), Tetrafluoromethane (CF4),
Isopropanol alcohol (IPA), cables, etc. Figure 1 shows part of a spectrum
(2700-3100 cm-1) from a smoldering fire of a regular extension cable (with a PVC
jacket). The evolution of HCl is evident, although the HCl band is overlapped
somewhat with a hydrocarbon band.
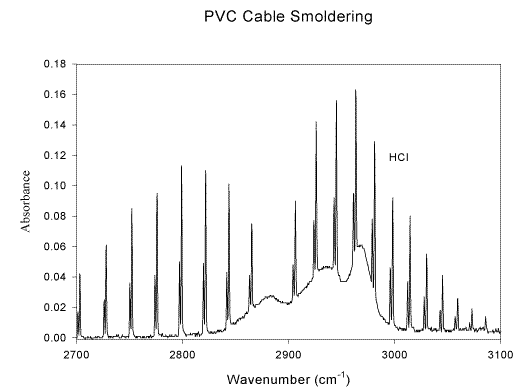
Figure 1 FT-IR spectral region indicating HCl evolution
from overheated wire cable
Figure 2 shows concentrations of some fuel specific species. N2O and
formaldehyde were clearly observed in a smoldering-flaming Douglas fir fire test
shown in the figure. Similar observations can be made for other materials
tested.
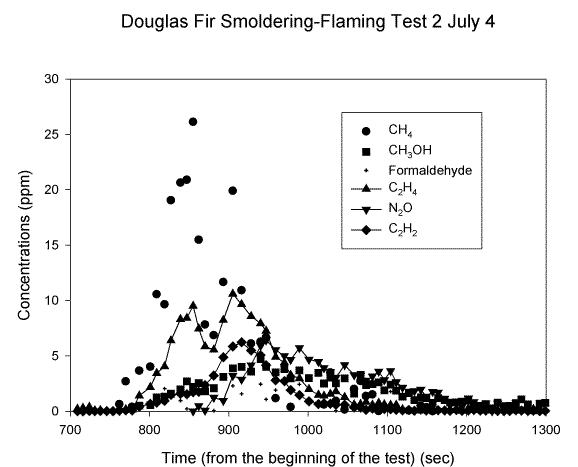
Figure 2 Gas concentration of Douglas fir fire test
The species concentrations measured by a FT-IR, together with a neural network
and fuzzy logic models, can be used to identify whether there is a fire or
nonfire (environmental/nuisance) event and to classify whether it is a flaming
or smoldering fire if the event is indeed a fire. A commercially available
neural network software package, NeuralWorks Professional II/Plus (), was chosen
to build the needed neural network. A so-called Learning Vector Quantization (LVQ)
network has been built and tested (Figure 3) The inputs to the network at this
moment are concentrations (18 species from FT-IR measurements) of CO2, CO, H2O,
CH4, CH3OH, Formaldehyde, HCl, C2H4, N2O, NH3, CF4, NO, Methyl Methacrylate,
Isopropanol alcohol, C2H6, C3H6, C6H14, C2H2, C6H6. The outputs of the network
are classification of the input data as a flaming fire, smoldering fire, or
nuisance/environmental source. The results were very successful, as among the
248 cases tested only 12 cases were misclassified, most due to the difficulties
in classifying the modes of combustion during a transition from smoldering to
flaming fire.
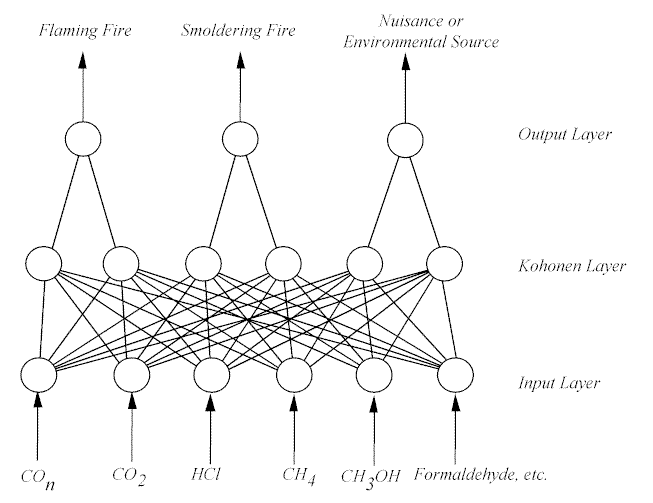
Figure 3 A Learning Vector Quantization (LVQ) network
to characterize fire and non-fire events.
We have incorporated the above-trained LVQ network into our data acquisition
system that connects with an On-Line 2010 multigas spectrometer. A real-time
fire detection system has been constructed. Preliminary tests of this integrated
software have been satisfactory using the test data we described above.
However, these tests are in no way rigorous, as we have only used data from a
single test arrangement. New tests (other burning materials, geometric
arrangement, etc.) are needed in order to validate the accuracy and improve the
robustness of the new fire detection. If you are interested in these techniques
or would like to become a commercial partner, please do not hesitate to contact
us.
- Milke, J.A. and McAvoy, T.J, Analysis of Signature Patterns for
Discriminating Fire Detection with Multiple Sensors, Fire Technology, Second
Quarter 1995.
- Serio, M.A., Bonanno, A.S., Knight, K.S., Wójtowicz, M.A. and Solomon,
P.R., Advanced Infrared Systems for Detection of Building Fires, Final
Report to DOC under Contract No. 50-DKNA-4-000-96, February 1995.
- Okayama, Y., Ito, T. and Sasaki, T., Design of Neural Net to Detect Early
Stage of Fire and Evaluation by Using Real Sensors’ Data, Fire Safety
Science-Proc. of 4th Int’l Symposium, pp. 751-759, 1993.
- 4 Okayama, Y., A Primitive Study of a Fire Detection Method Controlled by
Artificial Neural Net, Fire Safety Journal, pp. 535-553, 17, 1991.
- Chen, Y, Sathyamoorthy, Y, And Michael A. Serio, An Intelligent Fire
Detection System Using Advanced Infrared Diagnostics And Neural Network
Techniques, The Eastern States Meeting of the Combustion Institute, October,
1997.
- NeuralWare, Inc., NeuralWorks Professional II/Plus, Version 5.3, February
1997.
For more information contact mserio@AFRinc.com
|